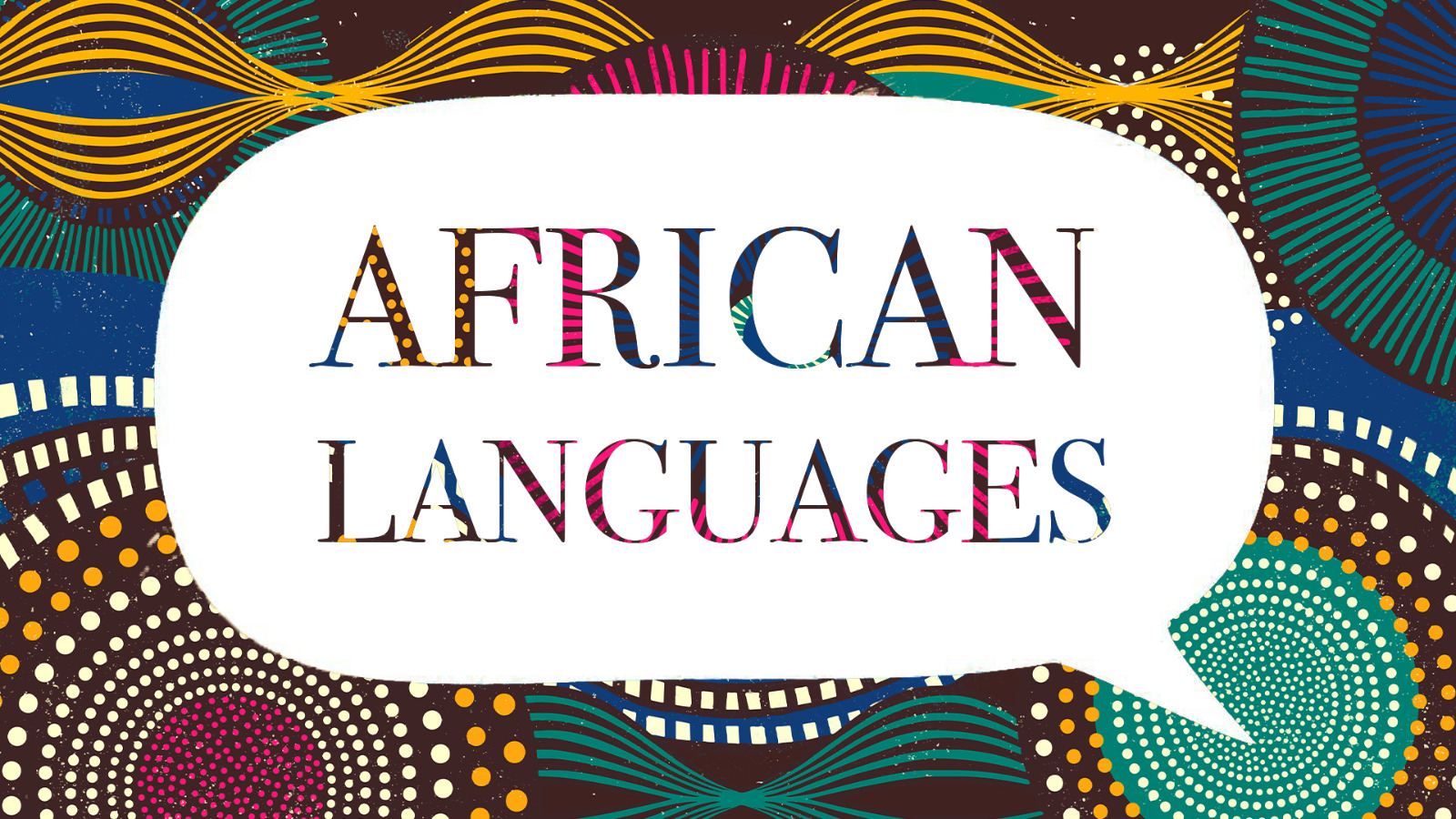
Lost in Translation: The digital struggles of African languages
Africa is home to rich linguistic diversity, with estimates suggesting that over 2,000 native languages are spoken across the continent.
Despite this diversity, many African languages are not supported by popular translation tools, including Google Translate, Microsoft Translate, and Translate, posing challenges for cross-border communication among millions of Africans.
While there has been significant progress in the field—such as Google’s 2024 addition of 110 new languages, about a quarter of which are from Africa—many challenges remain.
At the time, Google said the expansion aims to bridge the communication gap and provide better support for African language speakers.
However, users continue to encounter inaccuracies in translations and pronunciation. The complexity and nuances of African languages often make direct translation difficult, creating barriers.
“Dialectal variations can be so significant that some dialects are nearly distinct languages, further complicating translation efforts. Machine translation models also tend to perform better on high-resource languages, leaving low-resource African languages at a disadvantage,” said Evans Kofi Agyei, Project Manager at African Labs Language.
The organization specializes in providing Artificial Intelligence (AI) solutions that empower African languages.
It collects data on low-resourced African languages to establish comprehensive technological roadmaps and research methodologies.
“To optimize data availability and efficiency in training our machine translation (MT) models, we merge data from closely related dialects that exhibit high mutual intelligibility. For example, Asante Twi and Akuapem Twi are similar enough to be combined and processed as a single language. However, some dialects are distinct enough to warrant separate treatment as individual languages, such as Tshiluba and Kiluba,” he added.
Personal experiences highlighting the language barrier
A colleague at CGTN Africa from Nairobi, Kenya, who primarily speaks Swahili, said she encountered language barriers when she visited Ethiopia recently.
“I frequently had to rely on gestures or translation apps on my phone to communicate my needs, especially regarding transportation and food. This sometimes resulted in humorous misunderstandings, like ordering dishes I hadn’t meant to try or receiving unexpected items on my plate,” she described.
While local assistance was available, the lack of reliable translation tools posed challenges.
Similarly, as a South African residing in Kenya, I have faced difficulties with translation apps. The inaccuracies in these tools highlight the broader issue of African languages’ underrepresentation in technology.
Another colleague from Addis Ababa, a native Amharic speaker, shared his struggles after returning home from the West.
“When I visited home after 14 years, I struggled with language and relied on Google Translate, but found it difficult to get accurate translations or pronunciations. The voices in translation apps didn’t even sound like native speakers,” he said.
He described how tourists also face challenges, as most translation apps fail to accurately handle Amharic, leading to frequent miscommunications.
These experiences are common across the continent as more people travel across borders for new opportunities. This highlights the growing need for accurate and efficient language translation tools.
Expert insights on bridging the language gap
Experts emphasize the need for developing natural language processing (NLP) technologies tailored to African languages.
“Translation tools for African languages face numerous challenges, ranging from severe underrepresentation in NLP research to a lack of standardization. One of the most pressing issues is the limited availability of high-quality data, which hinders the development of accurate models,” Agyei said.
Collaborations between tech giants and African organizations are also underway. There is a call for community engagement involving native speakers, which would ensure that translation tools are accurate and culturally relevant.
“Bridging the gap in AI translation for African languages requires expanding high-quality parallel corpora through community-driven data collection, web scraping, and public domain texts,” he suggested.
“Low-resource model training techniques, such as transfer learning and fine-tuning multilingual models, are essential. Language-specific preprocessing, including custom normalization and morphological analysis, improves linguistic accuracy. Human-in-the-loop validation ensures quality through native speaker feedback, while multimodal approaches integrating text and speech enhance model comprehension.”